Generative AI has emerged as a transformative technology with a variety of applications across numerous industries, and the banking sector is no exception. By harnessing the power of generative AI, banks can unlock innovative solutions to deal with complex challenges and enhance operational efficiency.
This blog will offer an overview of some key applications of generative AI in banking, showcasing how this cutting-edge technology is revolutionizing traditional banking practices and paving the way for a more intelligent and data-driven future.
4 Main Use Cases of Generative AI in Banking
#1 Fraud Detection
Identifying unusual and deceptive transactions is among the functionalities of Generative AI within the banking sector. In a study, researchers assembled a dataset including fraudulent transactions and used it in a Generative Adversarial Network (GAN), a type of generative model rooted in deep neural networks, to generate synthetic fraudulent transactions.
The generated data was then compared with real transactions to evaluate the GAN’s capability to identify abnormal transactions. Ultimately, it became clear that using a GAN-enhanced training dataset for identifying abnormal transactions yielded superior results compared to using the unprocessed authentic dataset.
This study found out that training GANs for fraud detection purposes yielded favorable outcomes by fostering sensitivity post-training to detect unusual transactions. This holds significant implications for financial institutions dealing with vast volumes of transactions.
A Case Study on Fraud Detection Using Generative AI in Banking
Swedbank implemented GANs to identify fraudulent transactions. GANs were trained to differentiate legitimate and illegitimate transactions by analyzing their patterns and creating visual representations through graphs.
Nevertheless, the usage of GANs for fraud detection poses the risk of producing misguided results (refer to Figure 1), indicating the need for further enhancements.
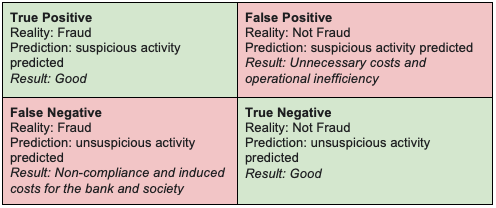
Figure 1. Confusion matrix of a financial fraud binary classifier.
#2 Risk Management
Establishing a risk management strategy is crucial for banks to maintain a suitable level of risk exposure, pinpoint potential risk areas, and take proactive measures to safeguard profitability. Inadequate handling of liquidity, credit, operational, and other risks can lead to financial losses.
In addition to using specific software systems geared aimed at risk mitigation, leveraging generative AI gives a viable solution for reducing such losses arising from inadequate risk management practices.
Using Generative Adversarial Networks (GANs), it becomes feasible to compute value-at-risk estimates, indicating the potential loss amount within a given timeframe, or to generate economic situations useful for forecasting the trajectory of economic markets. GANs aid in grasping the nuances of volatility by generating innovative scenarios devoid of assumptions, drawing insights from historic data patterns (Refer to Figure 2).
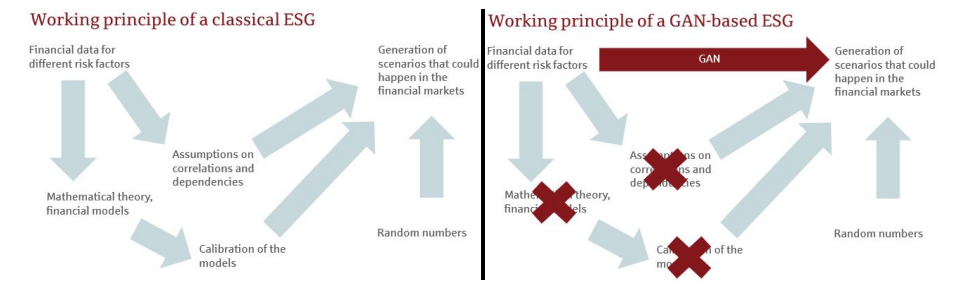
Figure 2. Comparison of the working principles of a classical and a GAN-based ESG
#3 Data Privacy
The adoption of synthetic data holds promise in addressing the challenges confronting the banking area, particularly concerning data privacy. Synthetic data offers a solution for generating substitute data that can be shared in lieu of customer data, which is restricted due to privacy regulations and data protection laws.
Additionally, synthetic customer data proves advantageous for training machine learning models to aid banks in assessing a consumer’s eligibility for credit or mortgage loans, as well as determining the appropriate loan amount.
Generative Adversarial Networks (GANs) stand out as an appropriate generation for producing synthetic data (Refer to Figure 3), making them well-suited to meet the requirements of the banking industry. Various versions of GAN, including Conditional GAN, WGAN, Deep Regret Analytic GAN, or TimeGAN, can be employed for synthetic data generation.
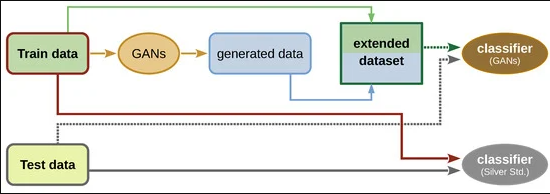
Figure 3: Schematic overview of the evaluation of data generated by GAN variants
#4 Creating Applicant-Friendly Denial Explanations
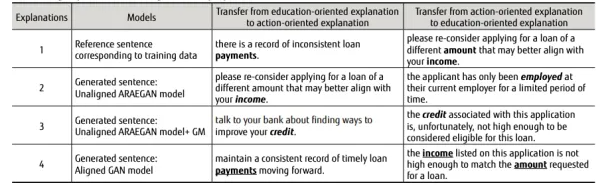
Figure 4: Generating explanations serving different purposes
AI plays an important role in various aspects of banking, including loan decisions. Through AI, banks can assess whether a customer qualifies for credit using risk-scoring techniques. Additionally, AI aids in making well-informed decisions regarding the maximum credit limit for a customer and determining loan pricing based on assessed risks.
However, it is not only decision-makers who require insights into AI-based decision-making processes; loan applicants also seek explanations, particularly when their applications are denied. Offering such explanations is essential not only to foster trust among users but also to enhance customer awareness, empowering them to make extra informed applications inside the future.
In this context, conditional GANs offer a solution. A conditional GAN, a variation of GAN where both the generator and discriminator are conditioned through class labels, proves useful for generating denial explanations that are user-friendly (Refer to Figure 4). By organizing the reasons for denial hierarchically, from simple to complex, a two-level conditioning approach is established to produce easily understandable explanations.
Working With an Experienced Partner For Your Software Product
Ready to enhance your development capacities or tackle intricate software projects? Reach out to our team today to discuss your objectives. Whether you’re looking to scale your team, explore emerging technologies, or accelerate your time-to-market, we have the knowledge and resources to support you.
Contact us to learn about how we can transform your software obstacles into success stories.